Deep Learning & MLOps Workflows
Amazing tools exist for the R&D/training phase (like wandb.ai). They let you create easily create experiments without worrying about version control, without to setup a projects, and they have features that QA-Board doesn't have (compare N training curves at the same time, early stopping, nice reports built-in...).
However, in our experience, tools built for training usually lack important features to evaluate inference:
- going in depth into specific difficult inputs (in our case images...): the training loss doesn't tell the whole picture
- comparing versus reference/previous versions
- giving users tools to trigger runs on new inputs (e.g. test database supplied by client)
- integration with source control, CI systems, etc
- rich/custom visualization
For instance here is how users can compare runs in QA-Board:


So how do we use QA-Board and deep learning tools? Well, we setup our projects to have them work well together.
Workflows
Since training is best done with dedicated tools, our projects are setup to show in QA-Board:
- the evaluation metrics
- model parameters and training summary
- link to the training page
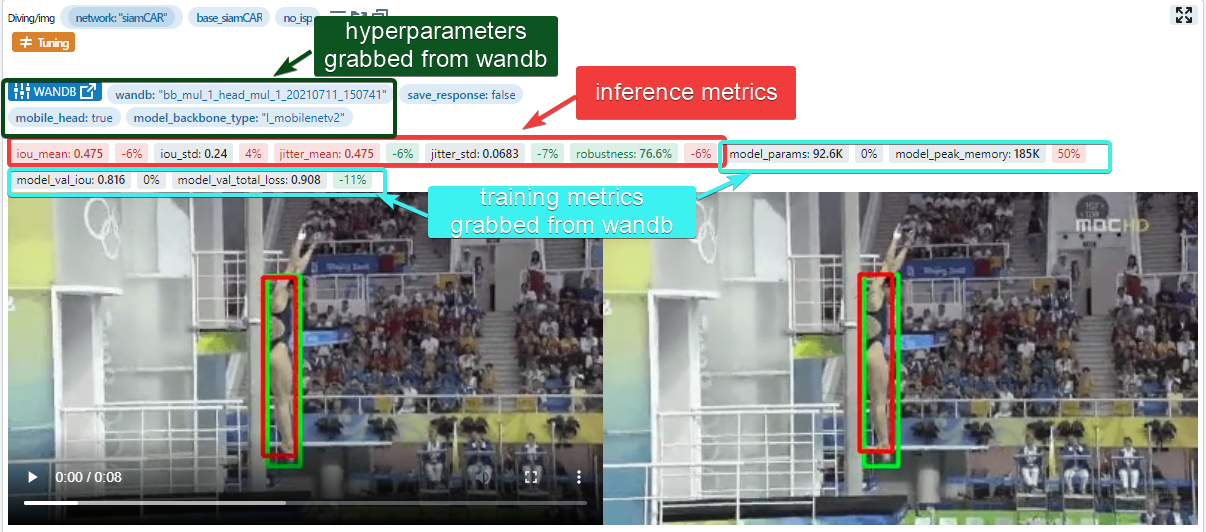
In practice:

Users get the best of both worlds: QA-Board, and the experiement/training-loss UI from wandb
...
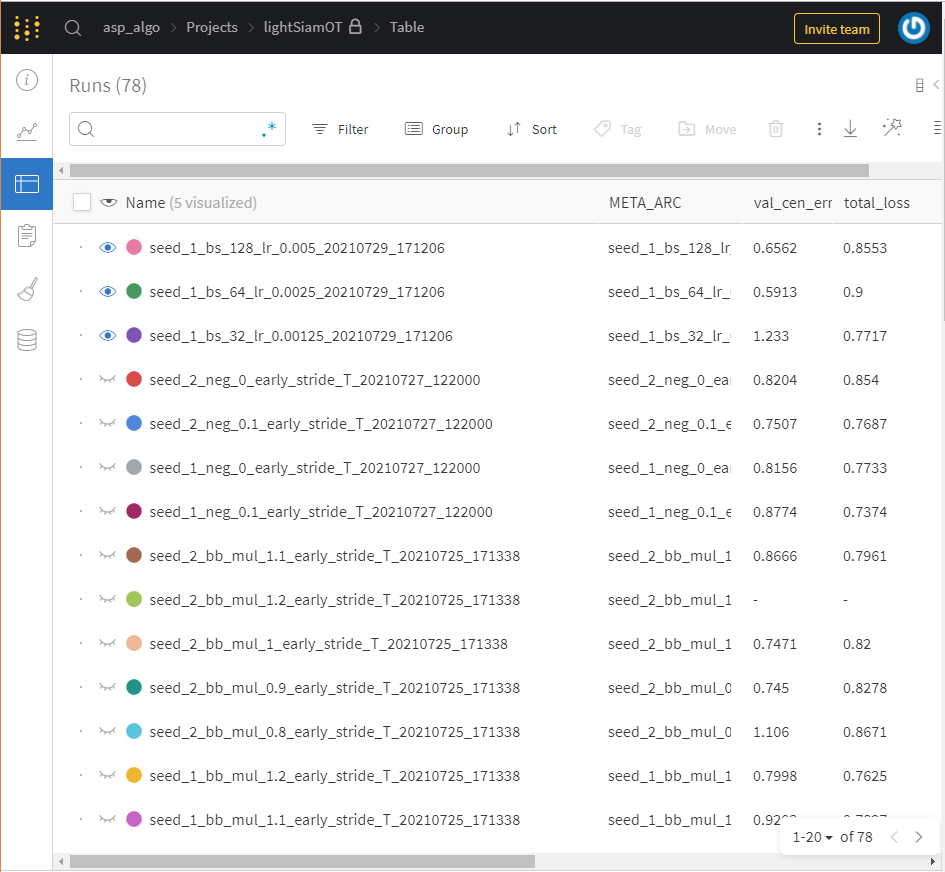
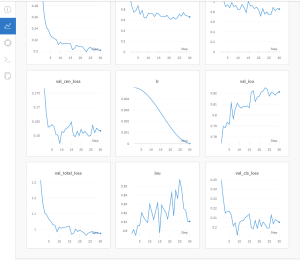
What is needed to make this work?
Users will write a run()
function that:
- Reads from
context.params
some model ID (or defaults to the model that was trained for the commit) - Fetches details about that model from some API: hyper-params, link to the training page... It will return those as badges
- Runs the model on the
context.input_path
- Does postprocessing to compute various evaluation metrics/plots

tip
Some engineers have a script that filters/sort their recent models according to various hyperparams/metrics, and start evaluation with QA-Board... Ideally we should be able to trigger directly inferences from the training page but not all ML tools make it easy!
MLOps
When using MLops flows, users will have the CI:
- Train a network
- Fail if the training loss is too high
- Run inferences on an evaluation batch using QA-Board
- Fail if the evaluation metrics are bad